Once this gene-by-gene approach has been explored, the PSB can then build upon gene level hypotheses, by identifying novel “digenic” (i.e. gene-gene) interactions that are present in the patient. VUStruct uses a machine learning approach (DiGePred1; github; web app). DiGePred was trained using a known database of digenic gene pairs (DIDA2) and gene pairs derived from unaffected relatives of individuals with rare undiagnosed diseases, part of the Vanderbilt Undiagnosed Diseases Network (UDN) cohort (Figure 1). Biochemical pathway similarity, phenotype annotation similarity, biological network proximity and other systems biology, genomics and evolutionary features were used to identify digenic pairs, and performance was validated on an external set of digenic pairs from recent literature, not included in DIDA.
The DiGePred analysis is a part of the PSB’s weekly variant effect summary report on rare variants identified in a UDN patient. This PSB approach has been undertaken within the Vanderbilt UDN cohort and over 100 clinical cases have been analyzed collaboratively, with some promising candidate variant combinations being currently experimentally validated. The DiGePred analysis was performed for a rare disease cohort of individuals with MRKH, and a novel digenic pair was identified3.
DiGePred analysis may identify gene pairs where co-disruption (as predicted by VUStruct) has occurred uniquely in the diseased individual, and may result in synergistic pathogenicity. As the volume of genomic and proteomic data continues to increase, we anticipate the importance of the DiGePred and PSB will become ever-more relevant to patient outcomes.
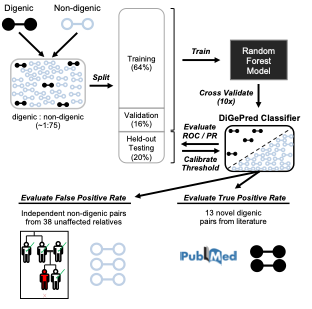
Figure 1: DiGePred trained on digenic pairs from Digenic Diseases Database (DIDA) and non-digenic pairs from unaffected relatives of UDN patients; evaluated in held-out sets and novel digenic pairs from recent literature.
References:
[1] Mukherjee, S., Cogan, J.D., Newman, J.H., Phillips, J.A., Hamid, R., Meiler, J., and Capra, J.A. (2021). Identifying digenic disease genes via machine learning in the Undiagnosed Diseases Network. Am. J. Hum. Genet. 108, 1946–1963.
[2] Gazzo, A.M., Daneels, D., Cilia, E., Bonduelle, M., Abramowicz, M., Van Dooren, S., Smits, G., and Lenaerts, T. (2016). DIDA: A curated and annotated digenic diseases database. Nucleic Acids Res. 44, D900–D907.
[3] Mikhael, S., Dugar, S., Morton, M., Chorich, L.P., Tam, K.B., Lossie, A.C., Kim, H.G., Knight, J., Taylor, H.S., Mukherjee, S., et al. (2021). Genetics of agenesis/hypoplasia of the uterus and vagina: narrowing down the number of candidate genes for Mayer–Rokitansky–Küster–Hauser Syndrome. Hum. Genet. 140,.